The Power of Bounding Box in Data Annotation Tool
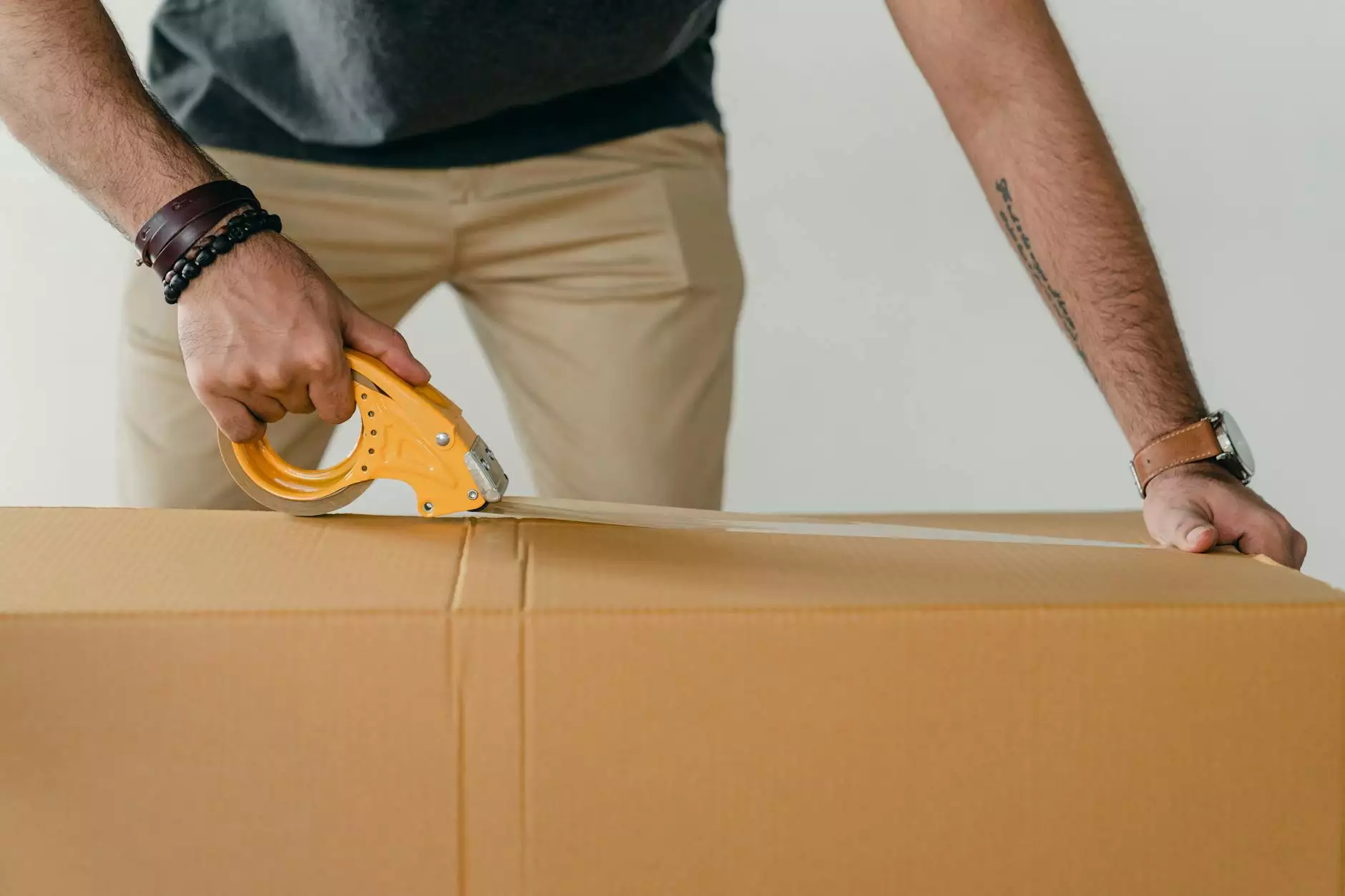
When it comes to efficient and accurate data annotation, the concept of bounding box plays a crucial role in the realm of AI and machine learning. At keylabs.ai, we specialize in providing cutting-edge solutions in the field of Data Annotation Tools and Data Annotation Platforms.
The Significance of Bounding Box
A bounding box is a rectangular border that outlines the shape of an object within an image or a frame. It is commonly used in object detection tasks to identify and localize specific objects within a larger visual context. Bounding boxes are essential in training computer vision models as they provide the necessary spatial information required for accurate recognition and classification.
Benefits of Using Bounding Box
- Accurate Object Detection: Bounding boxes help in precisely delineating objects of interest, enabling AI algorithms to detect and classify them with high precision.
- Enhanced Model Training: By providing clear boundaries around objects, bounding boxes aid in improving the performance of AI models during the training phase.
- Efficient Data Annotation: Annotation tools that incorporate bounding boxes streamline the annotation process, making it faster and more effective.
- Robust Object Localization: Bounding boxes enable AI systems to locate objects within an image, allowing for accurate spatial referencing.
Data Annotation Tools at keylabs.ai
At keylabs.ai, we offer state-of-the-art Data Annotation Tools that make use of advanced techniques, including bounding boxes, to facilitate precise labeling of datasets. Our tools are designed to optimize the annotation process and enhance the quality of training data for AI applications.
Features of Our Data Annotation Tools
- Bounding Box Annotation: Our tools support bounding box annotation for accurate object localization and identification.
- Customizable Labels: Users can customize labels and annotations based on specific project requirements.
- Collaborative Annotation: Multiple users can collaborate on annotating datasets in real-time, ensuring consistency and accuracy.
- Integration Capabilities: Our tools can be seamlessly integrated with existing AI pipelines and workflows.
Data Annotation Platforms for Seamless Workflow
For organizations looking to scale their data annotation processes, our Data Annotation Platforms provide a centralized solution for managing annotation tasks, tracking progress, and ensuring data quality. With features tailored to meet the diverse needs of AI projects, our platforms offer a comprehensive suite of tools for efficient data labeling.
Key Features of Our Data Annotation Platforms
- Project Management: Easily create and manage annotation projects with customizable settings and workflow templates.
- Quality Control: Implement quality assurance measures to maintain annotation accuracy and consistency across datasets.
- Scalability: Scale annotation tasks to accommodate large volumes of data while maintaining annotation quality and efficiency.
- API Support: Integrate our platform with third-party applications and AI frameworks through robust API support.
Conclusion
In conclusion, the use of bounding boxes in data annotation tools and platforms is essential for accurate object detection, model training, and spatial localization in AI applications. At keylabs.ai, we strive to deliver cutting-edge solutions that leverage the power of bounding boxes to enhance the efficiency and effectiveness of data annotation processes. Explore our range of Data Annotation Tools and Platforms to unlock the full potential of your AI projects.