Transforming Business with Labeling Tool Machine Learning
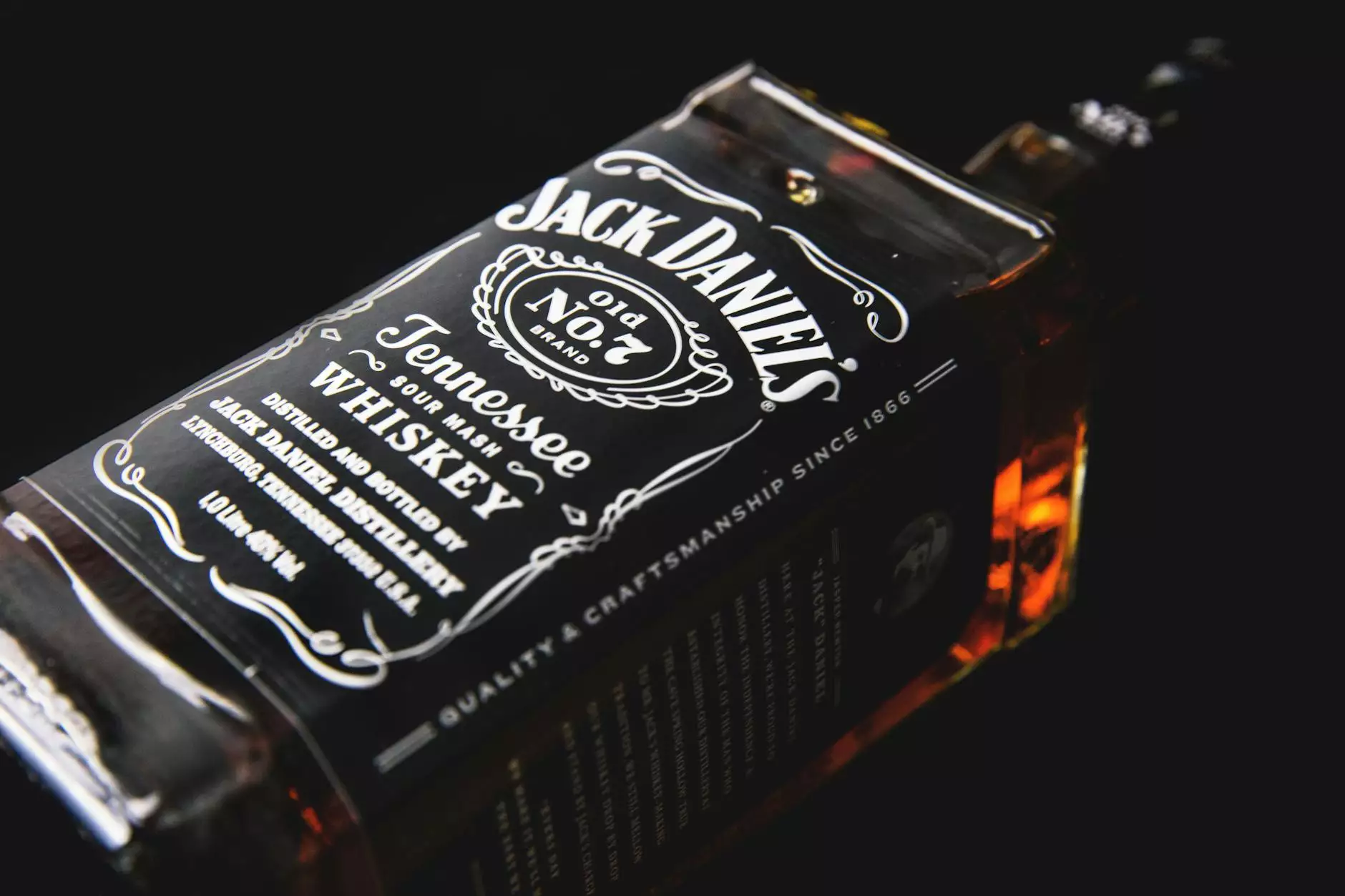
In today’s data-driven world, organizations are continuously challenged to enhance their decision-making processes through accurate data utilization. One of the forefront technologies making significant strides in this domain is the labeling tool machine learning. This technology not only advances the precision of data annotations but also streamlines processes, allowing businesses to focus on their core functions.
Understanding the Importance of Data Annotation
Data annotation is the process of labeling data to make it ready for machine learning algorithms. It is a crucial step as the quality of the annotated data directly impacts the efficiency and accuracy of any machine learning model. By using a reliable data annotation platform, businesses can ensure that their data is properly labeled, which is essential for producing effective predictive models.
Why Businesses Need to Invest in Data Annotation
- Enhancing Training Data Quality: High-quality labeled data is the backbone of machine learning. Without proper labels, models may underperform.
- Improving Decision Making: Accurate data leads to better insights, allowing businesses to make informed strategic choices.
- Speeding Up Development Cycles: A dedicated labeling tool can significantly reduce the time required for data preparation.
- Scaling Operations: Automated labeling processes enable businesses to manage larger datasets without sacrificing quality.
How Labeling Tool Machine Learning Works
At its core, a labeling tool machine learning utilizes sophisticated algorithms to automate the data annotation process. Here are some critical components of how this technology functions:
1. Input Data Collection
The first step involves gathering raw data, which could include images, audio, text, or video. This data serves as the foundation for the labeling process.
2. Machine Learning Models
Advanced machine learning algorithms are trained on previously annotated datasets. These models learn to recognize patterns and can make predictions on how new data should be labeled.
3. Annotation Process
Using the trained model, the labeling tool begins the annotation process, generating suggestions for how new data should be classified or labeled. Human oversight is still essential, ensuring transfer reliability.
4. Quality Assurance
Automated processes are supplemented with human verification. This dual approach ensures that the final output meets high-quality standards.
Advantages of Labeling Tool Machine Learning in Business
Implementing a labeling tool machine learning in your business practices can offer numerous advantages:
Enhanced Accuracy and Efficiency
With advanced machine learning models, the speed of annotation drastically improves while maintaining a high level of precision. Businesses can achieve remarkable efficiency in data processing tasks.
Cost-Effective Solutions
By reducing the amount of manual labor required for data annotation, companies can save on operational costs. Automated solutions provided by platforms like keylabs.ai help in reallocating resources more effectively.
Scalability
As your business grows, so does the volume of data. Implementing automated labeling tools allows organizations to effortlessly scale their operations without compromising accuracy.
Choosing the Right Data Annotation Tool
When searching for a data annotation tool, consider the following factors to ensure you select the best platform for your business needs:
- Type of Data Supported: Ensure the platform supports the types of data you work with, whether it be images, audio, video, or text.
- User-Friendly Interface: A tool with an intuitive interface will make it easier for your team to adopt and utilize effectively.
- Integration Capabilities: Choose a tool that easily integrates with your existing workflows and software solutions.
- Customer Support: Exceptional customer support can help troubleshoot issues quickly, minimizing downtime.
Best Practices for Data Annotation Using Machine Learning
Here are several best practices to implement when using a labeling tool machine learning:
1. Establish Clear Guidelines
Define the objectives and requirements for data annotation clearly. This ensures everyone involved interprets and labels data consistently.
2. Use a Combination of Automation and Human Oversight
While automation speeds up the process, human verification remains vital for ensuring data quality. Strike a balance between technology and manual review.
3. Continuously Update Your Models
Machine learning models can drift over time. Regularly updating and retraining your models based on new annotated data is crucial for maintaining accuracy.
4. Regularly Monitor and Audit Annotations
Conduct periodic checks to evaluate the quality of annotations. This helps identify areas for improvement and enhances the labeling workflow.
Future of Machine Learning and Data Annotation
As machine learning technologies continue to evolve, so too will the labeling tool machine learning. Businesses that leverage these advancements will enjoy competitive advantages through improved data handling capabilities. Emerging technologies such as AI-driven annotation and active learning are poised to further enhance automation in this space.
AI-Driven Annotation
AI-powered tools will revolutionize data annotation, allowing for quicker, more accurate labeling processes without extensive human intervention.
Active Learning Models
Active learning models will enable machines to learn from human feedback continuously, resulting in systems that become more intelligent and efficient over time.
Conclusion
The integration of labeling tool machine learning into your business processes is not merely a trend but a necessary evolution in the age of data. By embracing this technology, organizations can enhance their data annotation practices—boosting accuracy, reducing costs, and facilitating faster, data-driven decisions. Platforms like keylabs.ai offer robust solutions tailored to meet the ever-growing demands of data-centric businesses, ensuring that you remain at the forefront of your industry.
Frequently Asked Questions (FAQs)
What is a labeling tool in machine learning?
A labeling tool in machine learning is a software that assists in the data annotation process. It helps categorize and label data for training machine learning models.
Why is data annotation crucial for machine learning?
Data annotation is crucial as it prepares high-quality datasets that machine learning models need to learn from. Inaccurate labels can lead to poor model performance.
Can automated labeling tools replace human annotators?
While automated tools can significantly speed up the process and handle large volumes of data, human oversight is still essential to ensure the quality and accuracy of annotations.
How do I choose the best data annotation platform?
When choosing a platform, consider the types of data supported, integration capabilities, user-friendliness, and the level of customer support offered.
What are the trends in data annotation technology?
Current trends include the use of AI-driven annotation tools, active learning models, and the increasing importance of collaboration between machines and human annotators.