Unlocking the Power of Data: The Importance of an Annotation Tool for Image Segmentation
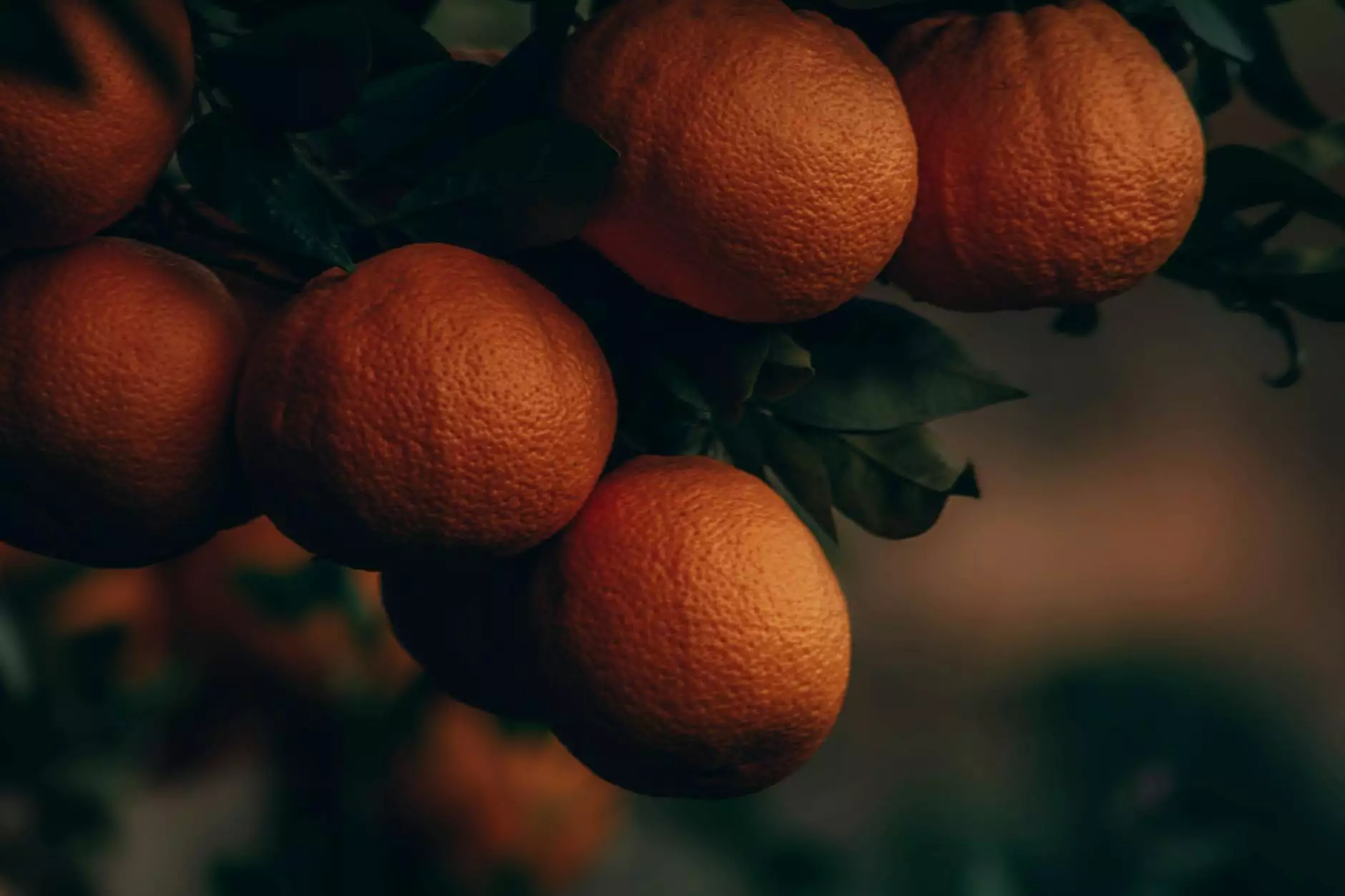
In today’s data-driven world, businesses and researchers increasingly rely on robust and sophisticated data annotation tools. One of the most crucial areas where these tools shine is in image segmentation. This process, essential for training machine learning models, enables systems to understand images at a granular level. Using an annotation tool for image segmentation not only streamlines the workflow but also significantly enhances the accuracy of the resulting data.
Understanding Image Segmentation
Image segmentation is a vital step in computer vision tasks, allowing machines to interpret images in a way similar to human perception. The objective is to partition an image into multiple segments or regions, making it easier to identify and analyze objects within the image. This process can be categorized into various types, including:
- Semantic Segmentation: Classifies each pixel in the image into a category for understanding the scene.
- Instance Segmentation: Differentiates between different objects of the same class.
- Panoptic Segmentation: Combines semantic and instance segmentation to provide a holistic view.
Each of these segmentation types serves distinct purposes and applications across different industries, making the choice of an effective annotation tool critical.
The Role of an Annotation Tool for Image Segmentation
An annotation tool for image segmentation serves several key functions that facilitate the image annotation process:
1. Enhanced Accuracy
Among the primary functions of an annotation tool is to allow for high precision in the segmentation of images. Automated features, such as polygonal segmentation, significantly reduce human error when defining image boundaries, leading to cleaner datasets.
2. User-Friendly Interfaces
Modern annotation tools often boast intuitive interfaces that simplify the segmentation process. Users can quickly learn the tools without extensive training, leading to improvements in productivity and efficiency.
3. Collaboration and Workflow Management
Effective annotation tools allow multiple team members to collaborate in real-time, fostering an environment of productivity. Built-in project management features enable teams to track progress and review work seamlessly.
4. Integration Capabilities
Most annotation tools can easily integrate with other platforms and tools in the AI development pipeline. This connectivity allows for streamlined workflows, enhancing the overall development process.
The Benefits of Using a High-Quality Annotation Tool
Investing in a quality annotation tool for image segmentation has far-reaching benefits that go beyond just enhanced productivity:
1. Cost-Effective Solutions
By reducing the time spent on manual annotation and enhancing the quality of the annotated data, businesses can achieve a better return on investment. The automation features offered by advanced tools reduce labor costs associated with manual data annotation.
2. Scalability
As your data needs grow, so does the requirement for more comprehensive annotation. A robust annotation tool can scale to handle increasing amounts of data without sacrificing quality or efficiency.
3. Continuous Improvement of AI Models
Quality annotated data is foundational for training machine learning models. The better the quality of the data, the more accurate the AI's predictions will be. Using a sophisticated annotation tool ensures that your AI applications are built on a solid foundation, substantially improving their performance.
Choosing the Right Annotation Tool for Image Segmentation
When selecting an annotation tool for image segmentation, consider the following factors:
1. Features and Functionality
Look for tools that offer a comprehensive suite of features including various segmentation options, automated labeling, and real-time collaboration capabilities. The more functionality, the easier it is to meet diverse project needs.
2. User Experience
An intuitive interface is crucial. Tools that are easy to navigate will reduce the onboarding time for new users and minimize errors in the segmentation process.
3. Support and Documentation
Quality support and thorough documentation can make a significant difference, particularly if you encounter challenges using the tool. Ensure that the provider offers strong customer support and extensive resources to aid users.
4. Cost
Evaluate the pricing models to ensure you are getting good value for your investment. Some tools may offer tiered pricing based on features, while others may have a flat rate. Choose one that aligns with your budget and needs.
Case Studies: Success Stories with Image Segmentation Tools
Various organizations have harnessed the power of annotation tools for image segmentation to propel their projects forward. Here are a few notable examples:
1. Autonomous Vehicles
Companies in the autonomous vehicle space utilize sophisticated annotation tools to segment images taken from vehicle cameras. These tools help differentiate between various objects on the road, such as pedestrians, other vehicles, and obstacles, thereby enhancing safety and reliability.
2. Medical Imaging
In the medical field, image segmentation is crucial for identifying pathological conditions in imaging studies. Machine learning models trained on high-quality annotated datasets can assist radiologists by providing preliminary assessments, thereby accelerating diagnosis and improving patient outcomes.
3. Agricultural Innovations
Agricultural technologies use image segmentation to monitor crop health through drone imagery. Annotations help identify disease symptoms in plants, allowing farmers to make data-driven decisions on treatment and resource allocation.
Future Trends in Image Segmentation and Data Annotation
The advancement of AI and machine learning technologies will continue to influence how data annotation is performed. Upcoming trends may include:
1. AI-Assisted Annotation
Future tools are likely to incorporate AI techniques to assist in the annotation process. This could speed up workflows through automated suggestions, enhancing efficiency and accuracy.
2. Expansion of Annotation Types
As computer vision applications evolve, the complexity and types of annotations needed will grow. Tools will be expected to adapt to these needs, offering more sophisticated options for users.
3. Enhanced Collaboration Features
The demand for remote collaboration has surged, requiring tools that facilitate real-time annotations across global teams. Enhanced collaboration features will be essential in the future.
Conclusion
The integration of a reliable annotation tool for image segmentation into your data processes is not just beneficial; it’s essential for staying competitive in an increasingly data-centric marketplace. By investing in advanced annotation tools, businesses can significantly enhance the quality of their datasets, optimize workflows, and foster innovation in AI applications. Embrace this exciting opportunity and unlock the potential that high-quality image segmentation has to offer.